Research
Deep learning is not easy. For example, applying deep learning on a specifcal microscopy image analysis problem is much more than collecting images, making annotations, and feeding the images with annotations into a neural network. On one hand, annotation is not easy and sometimes even impossible (e.g., accurately annotating the segmentation of a very complex shape in 3D is nearly impossible). On the other hand, there are many important questions to think about. How to evaluate the quality of your annotation? What if different people have different annotations? How can I annotate the minimal amount of data to achieve to desired accuracy? When I apply my model on new images, how do I know the results are correct? …
Solving real computational biomedical problems with AI is not straightforward. The quantitative tasks in many biomedical applications may not be easily formulated into common deep learning or computer vision problems (e.g., segmentation, classification, image reconstruction, etc.). Even a well-defined problem, we may face very different challenges in the specific biomedical context than the problem’s original general computer vision setting. For example, it would be easy for everyone to accuratly annotate the contour of a car in a 2D image, but it could be very difficult to annotate the boundary of a nucleus in a 3D confocal microscopy image. It is not only because 3D is hard, but also because you have to take the diffraction of light into account to avoid over-segmentation. Or, minor segmentation error of cars may not be a big deal, but minor segmentation error of nuclei (e.g., over-smoothed boundary of nuclei with complex shape when being squeezed) may yield very different biomedical findings. So, to develop a successful AI-based microscopy image analysis algorithm, it is very important to have good understanding of microscopy and the underlying biomedical application.
2025
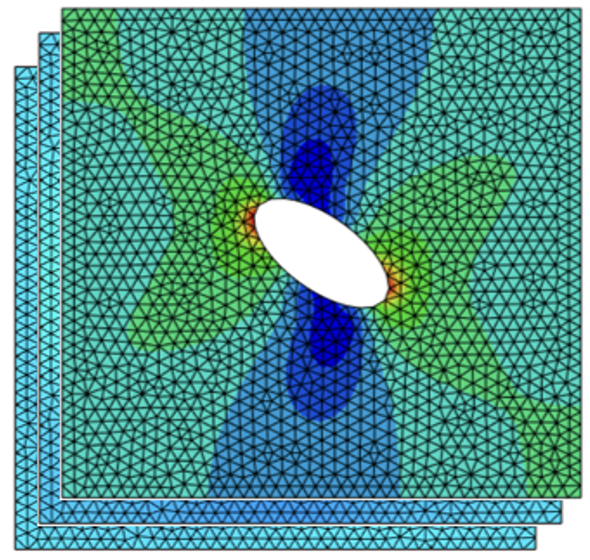
2024
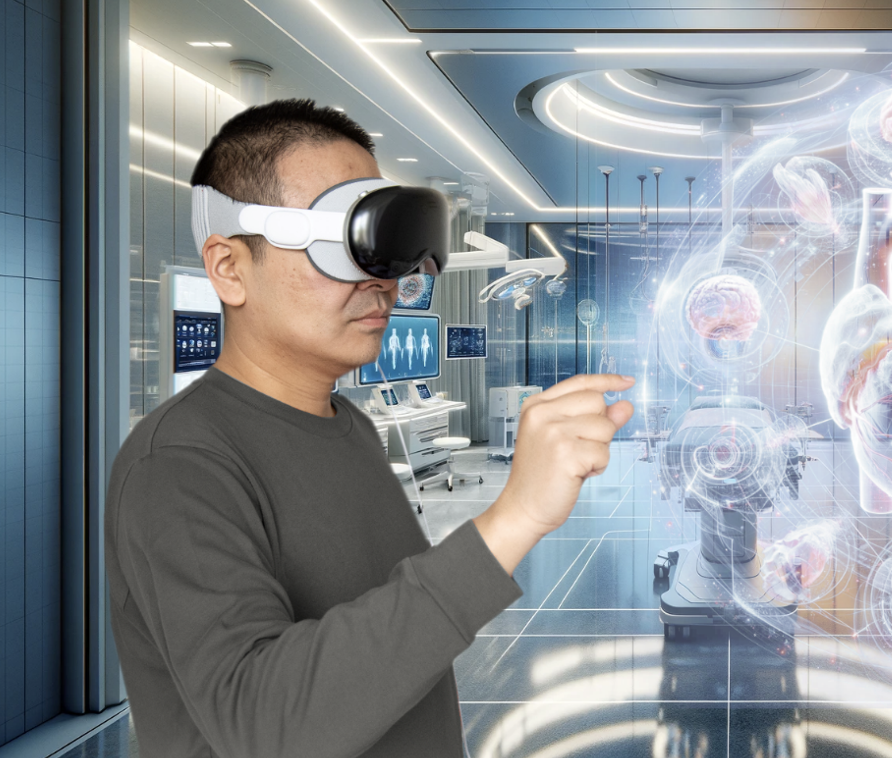
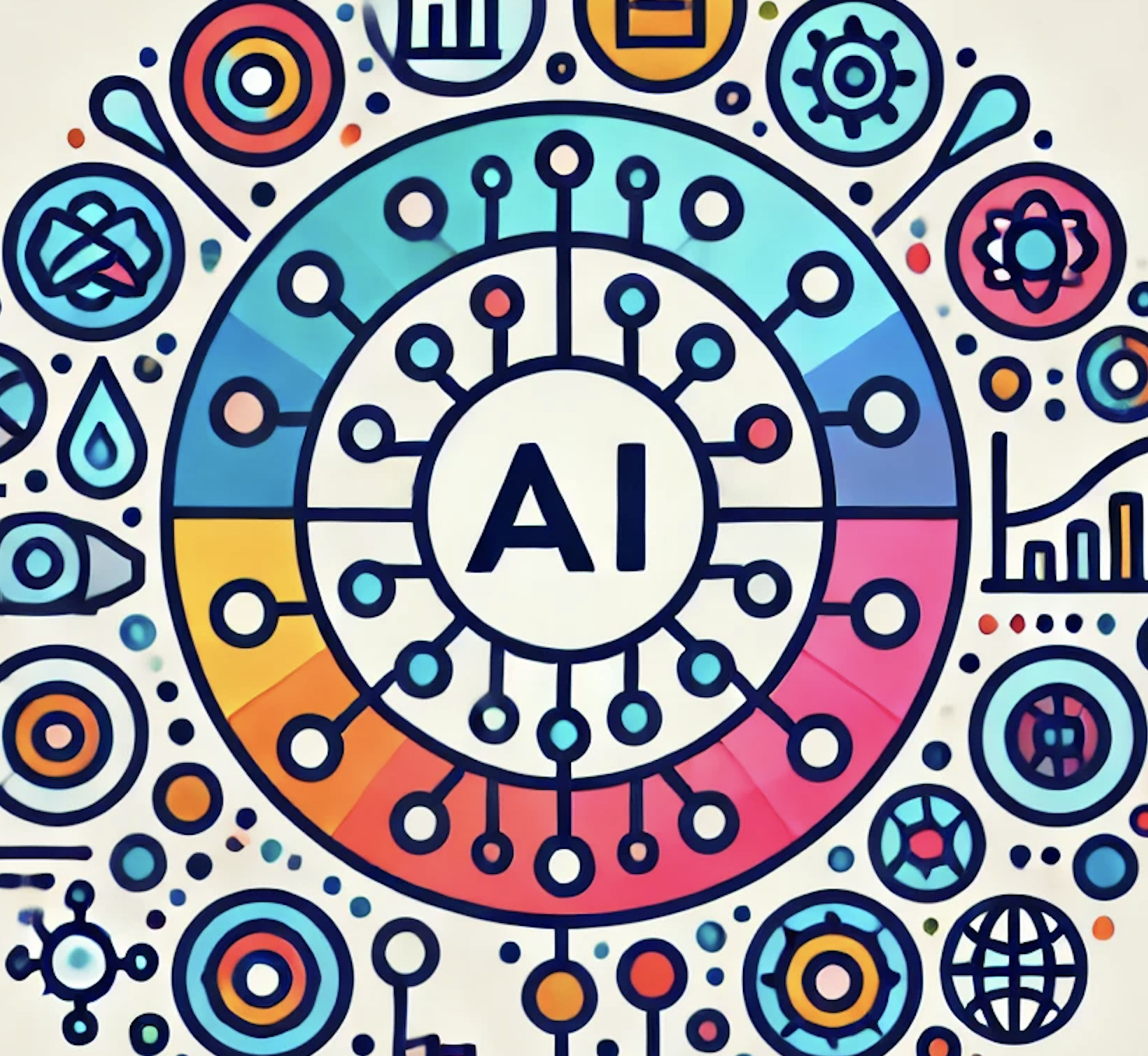
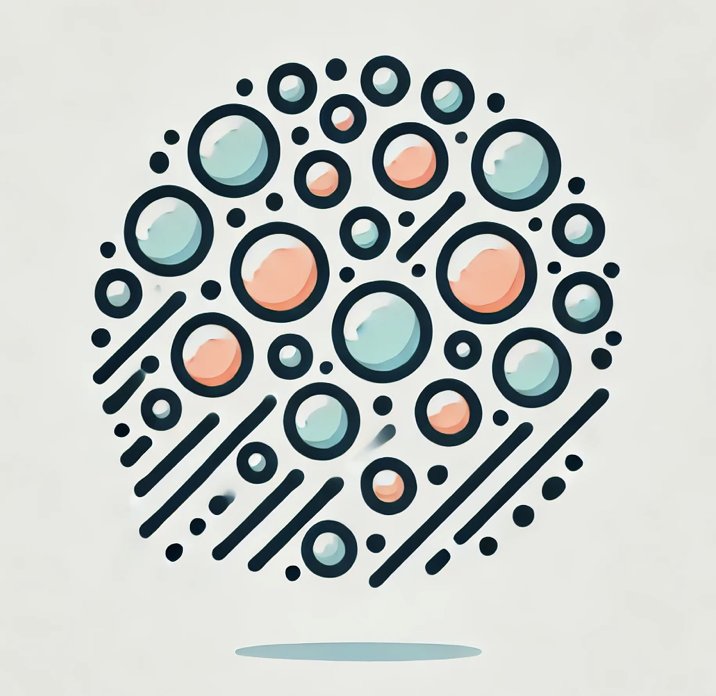
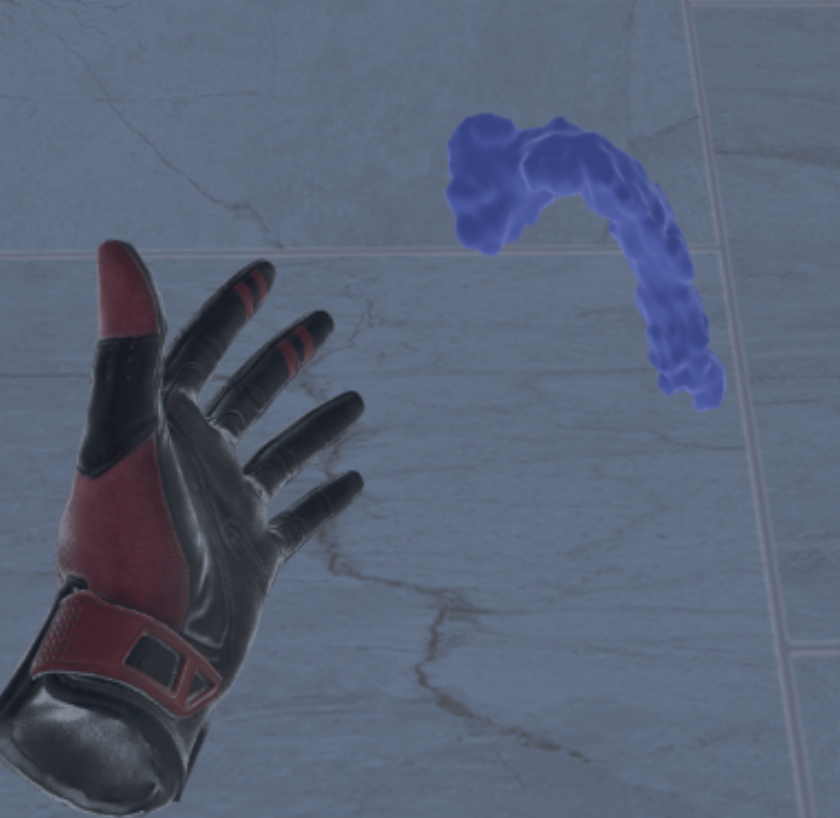
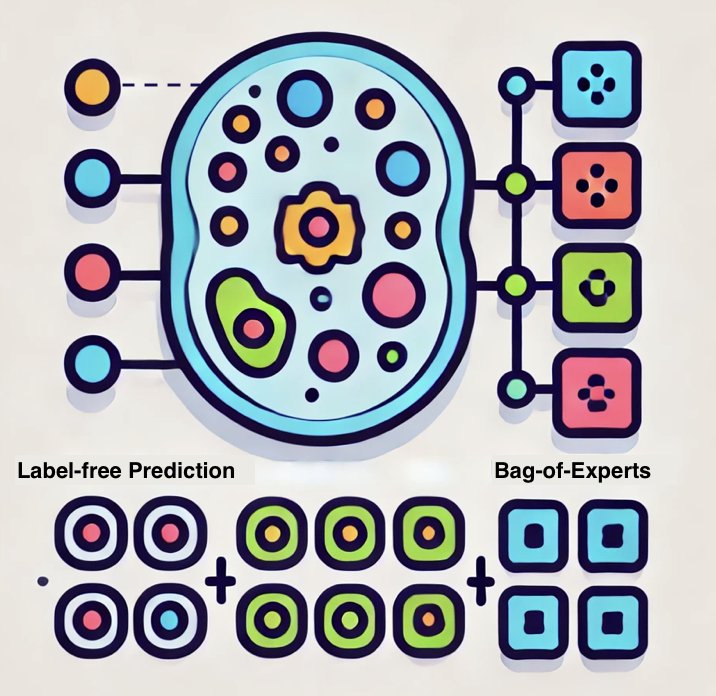
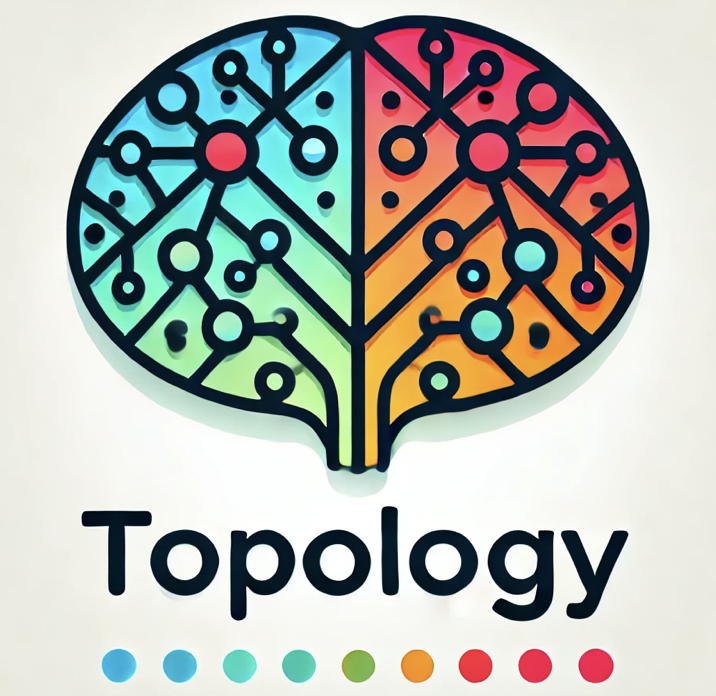
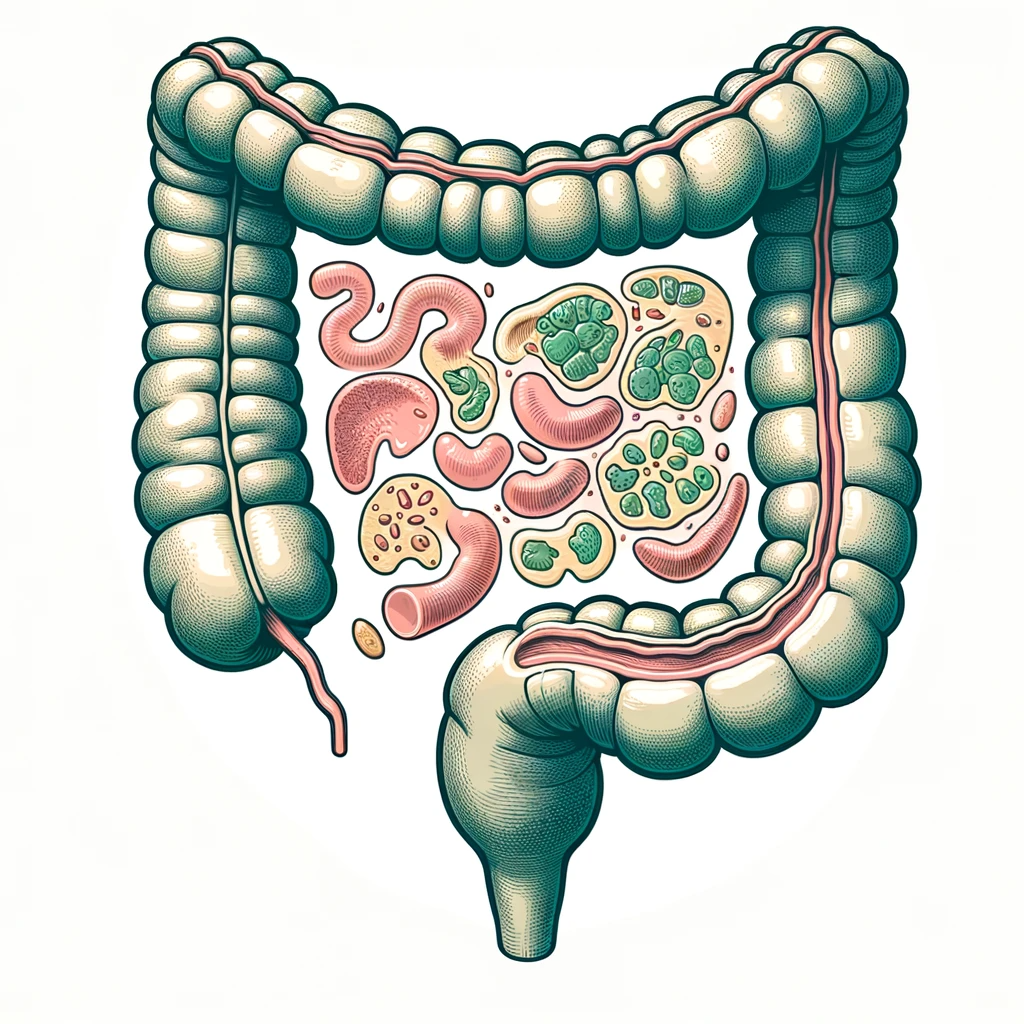
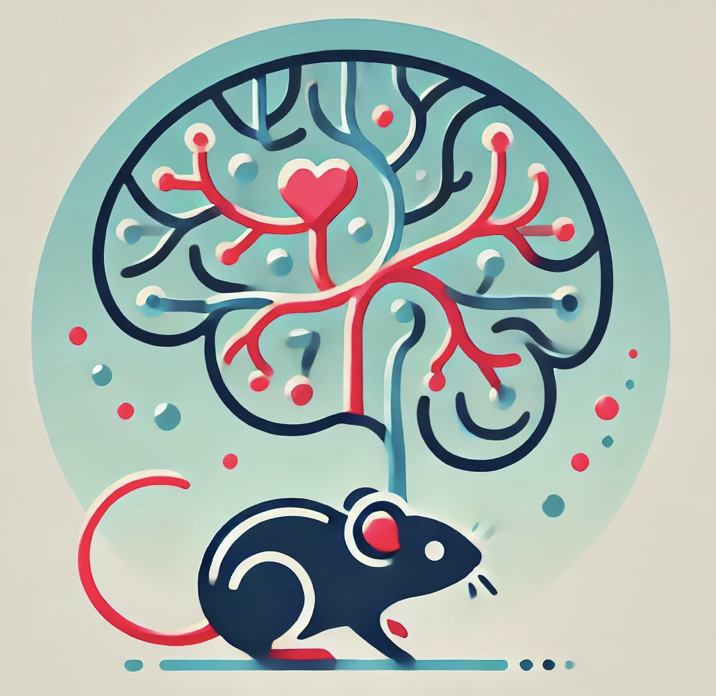
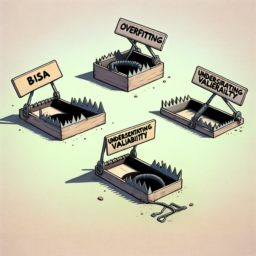
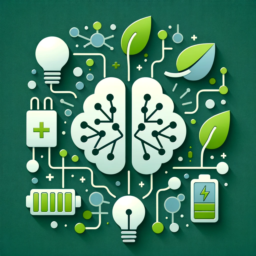
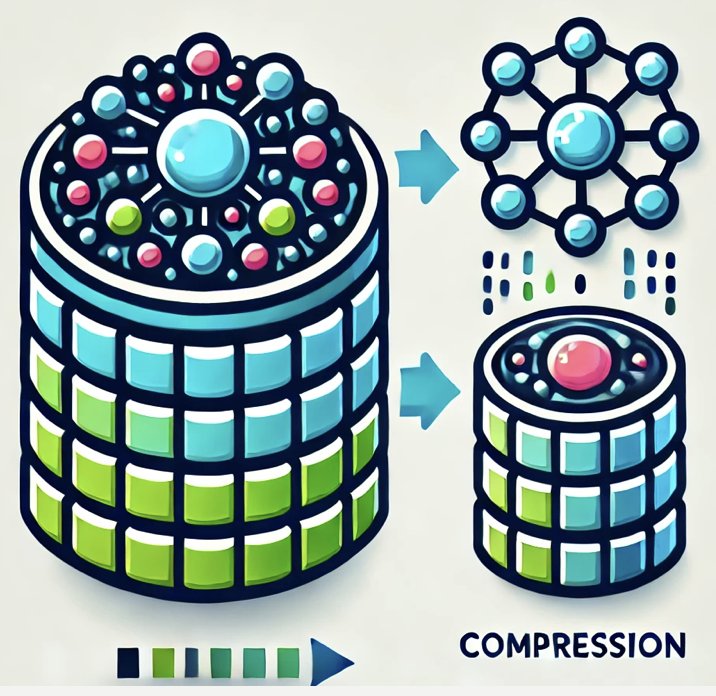
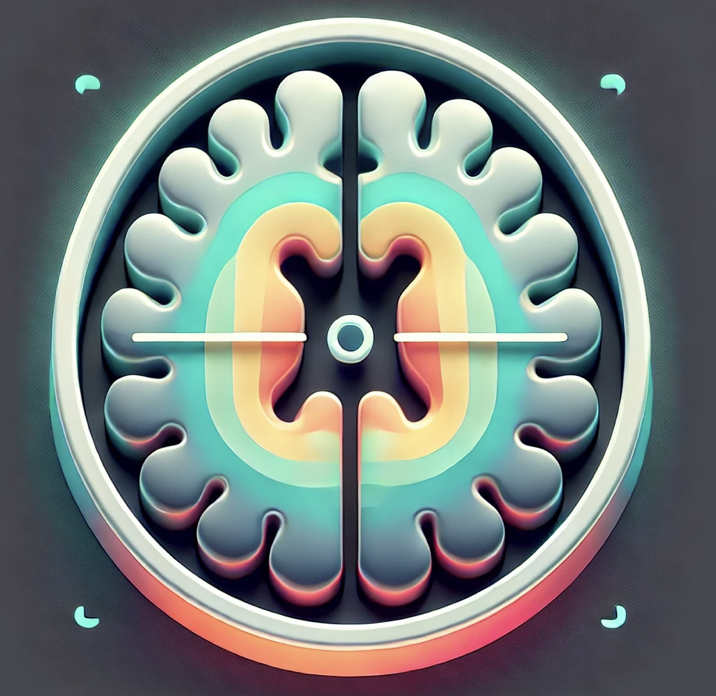
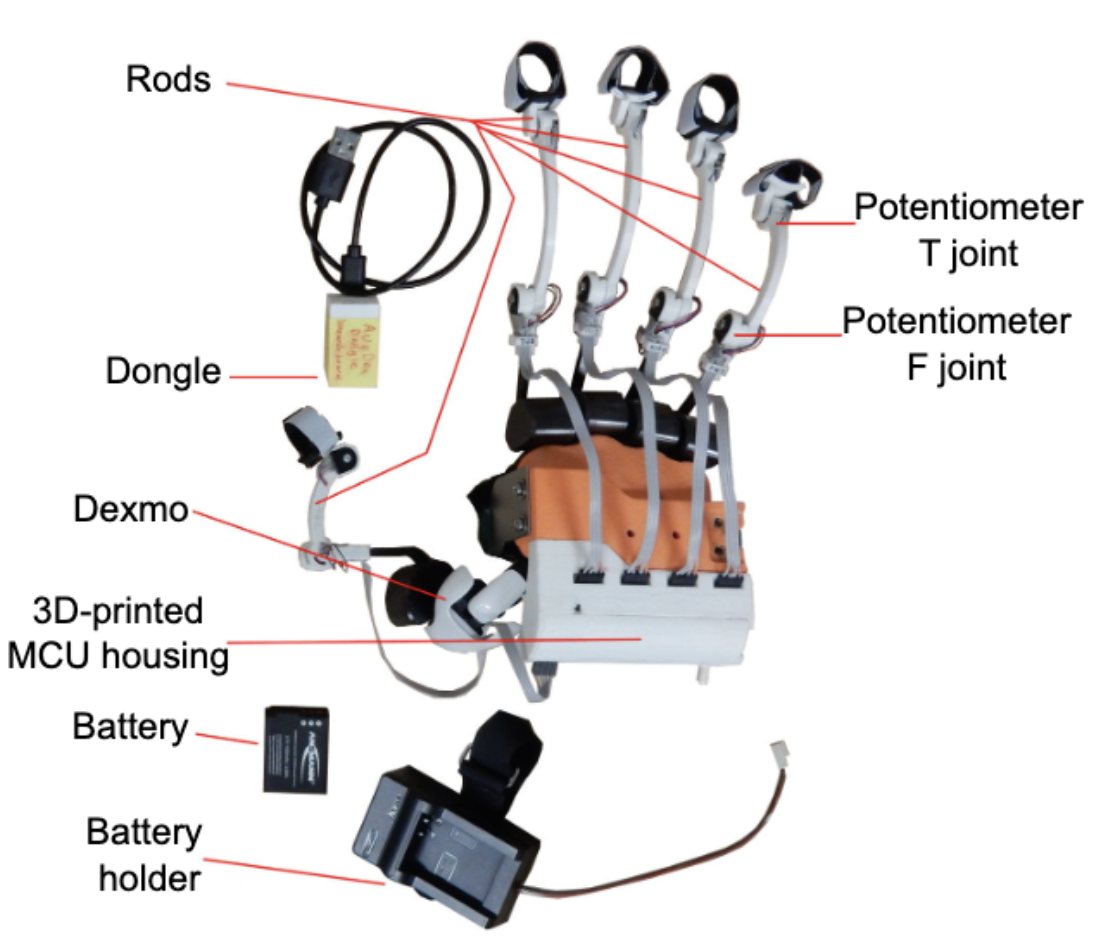
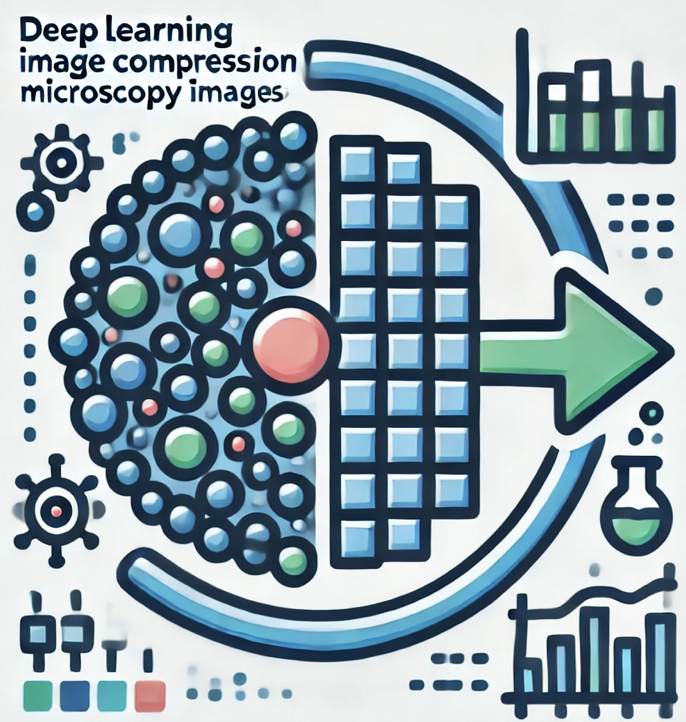
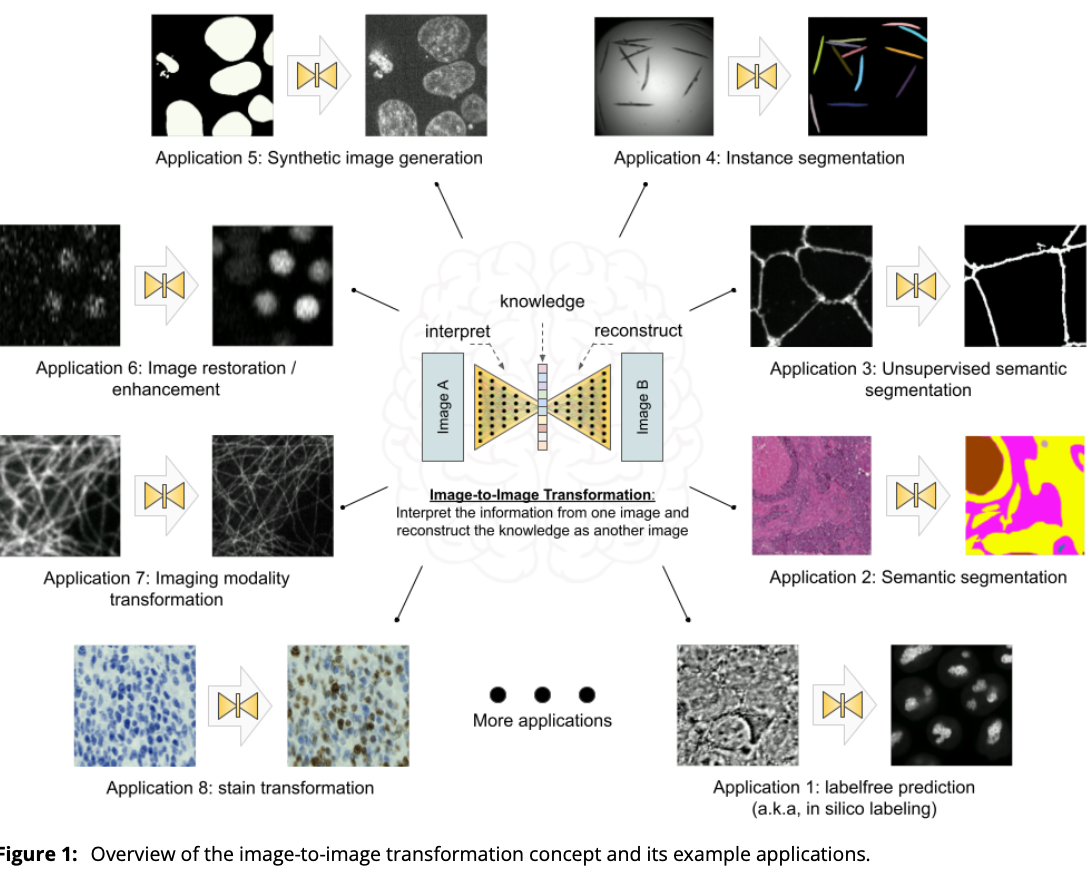
2023
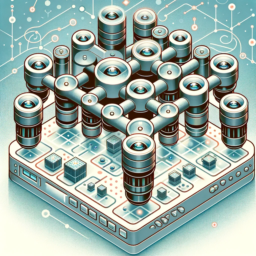
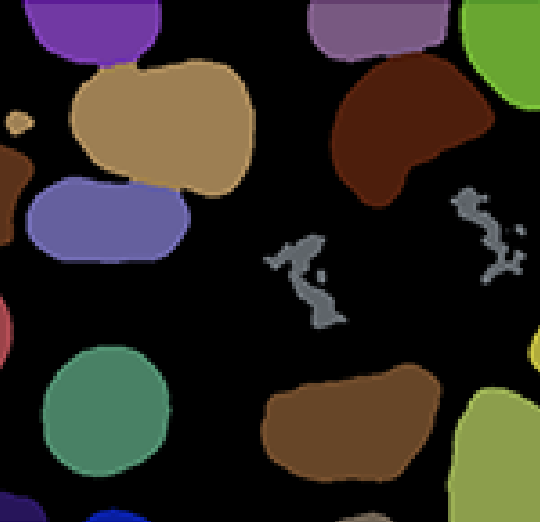
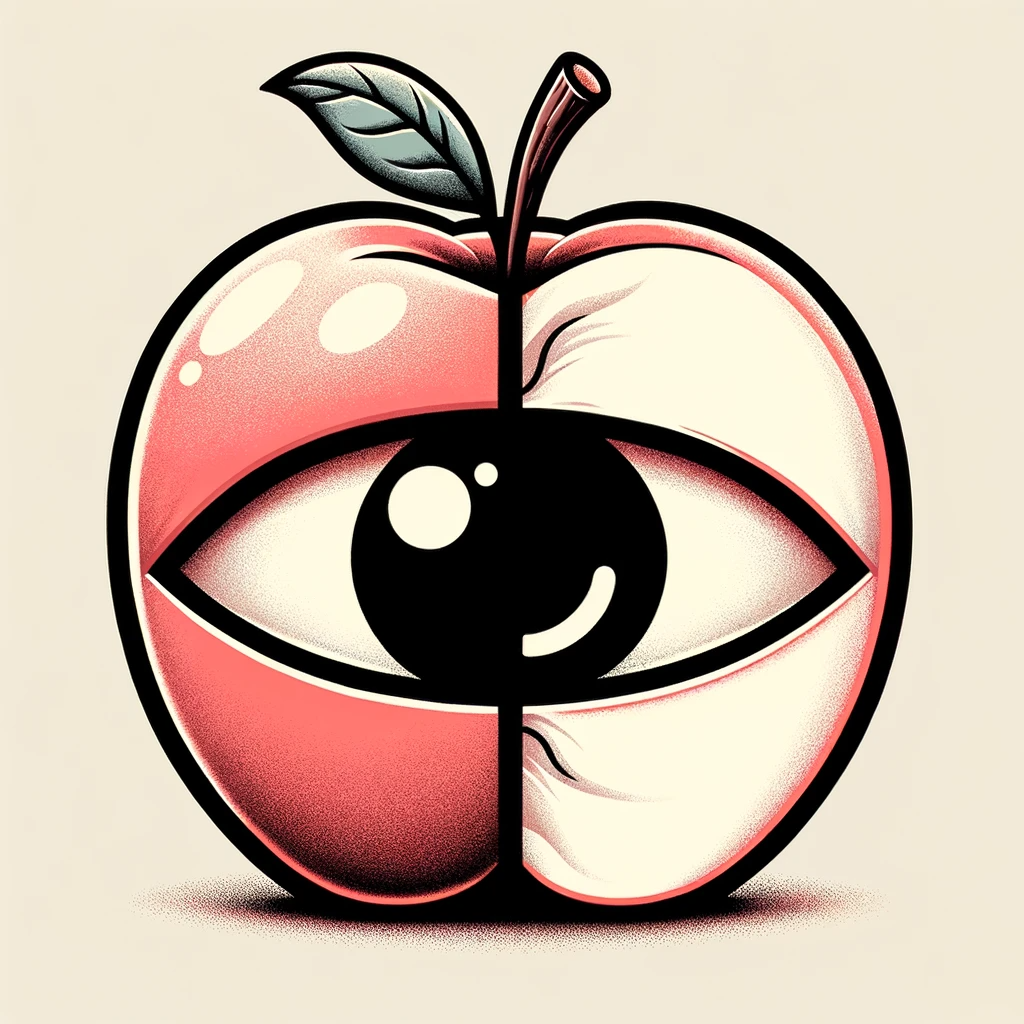
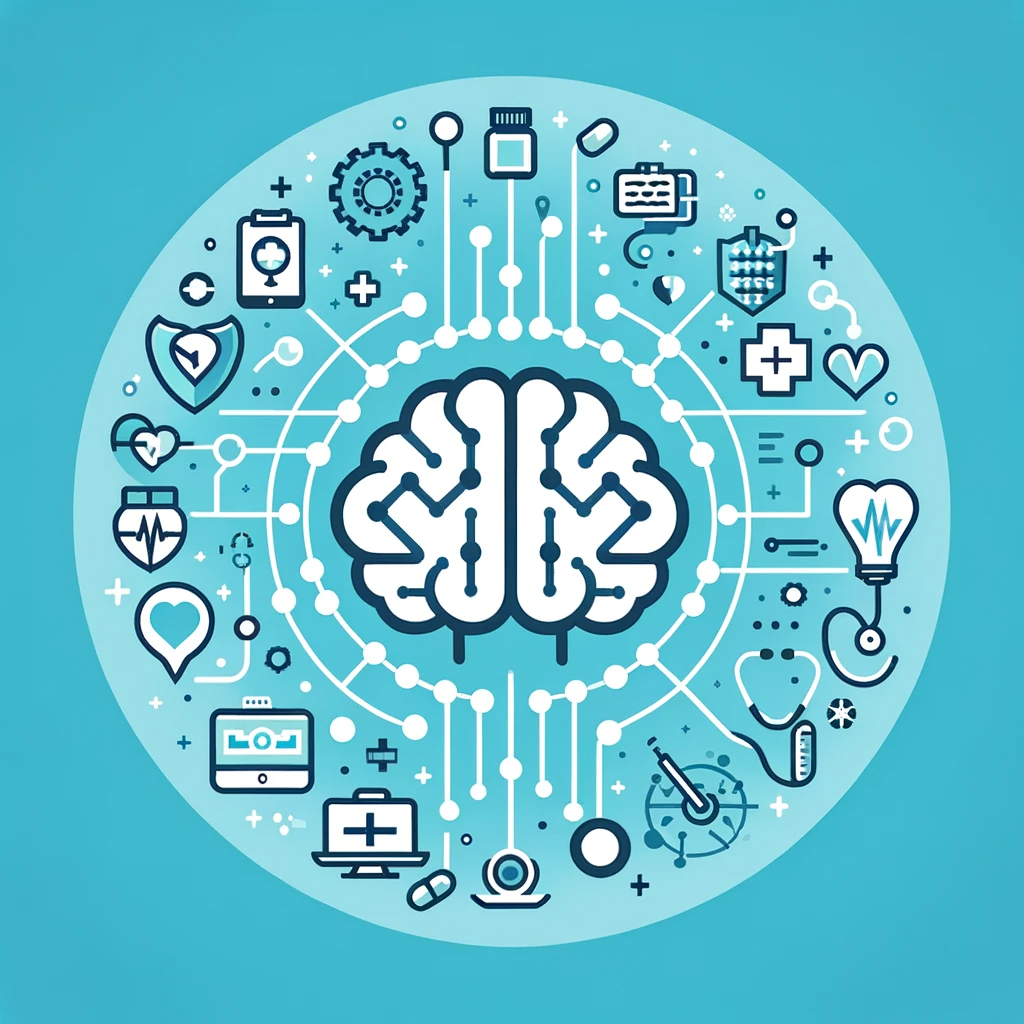
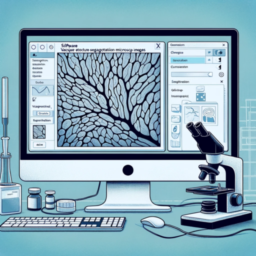
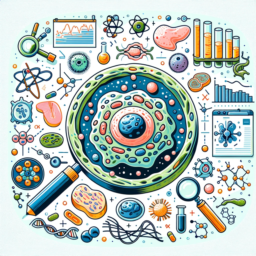
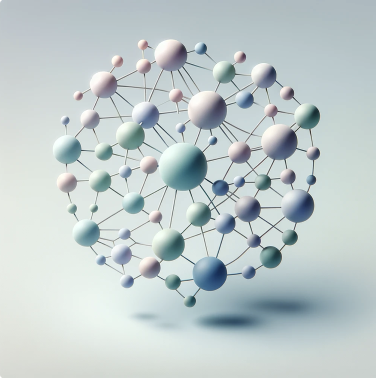
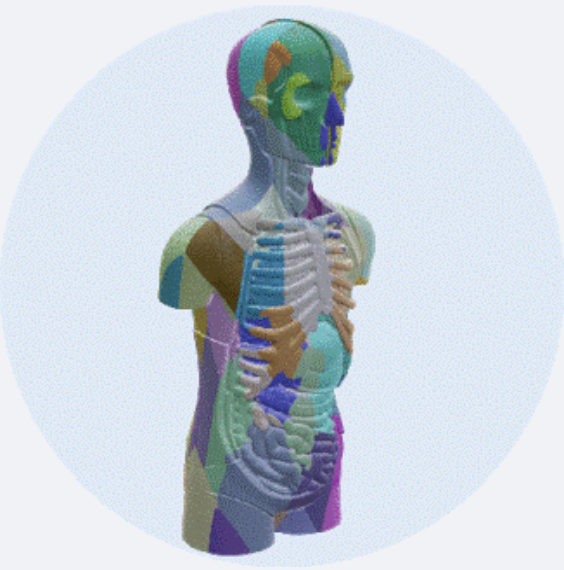
2022
2021

2020
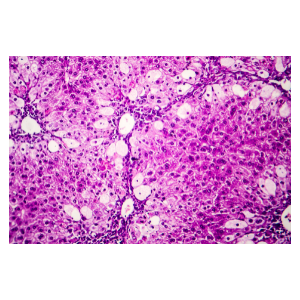
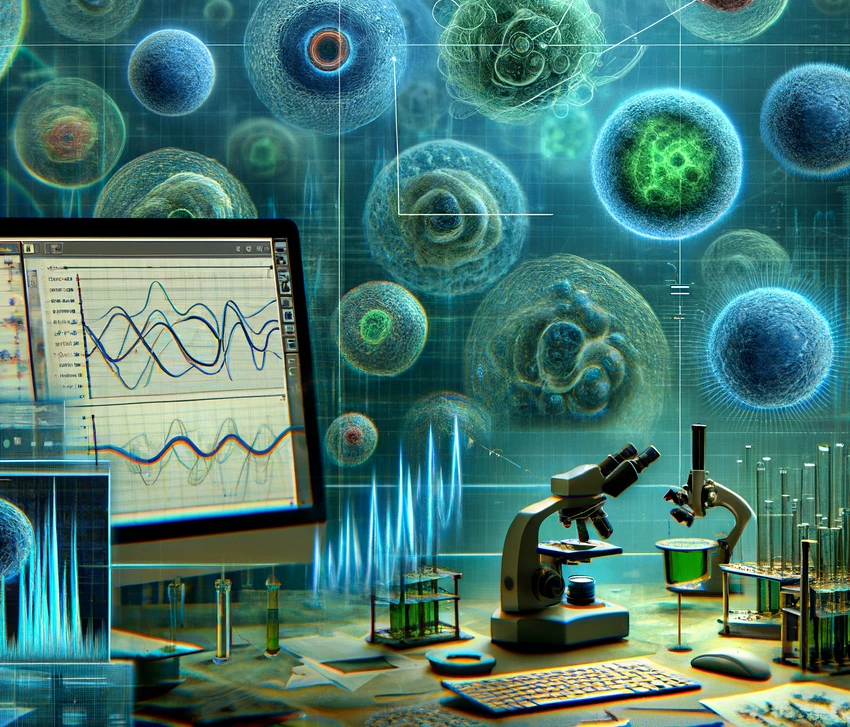
2019
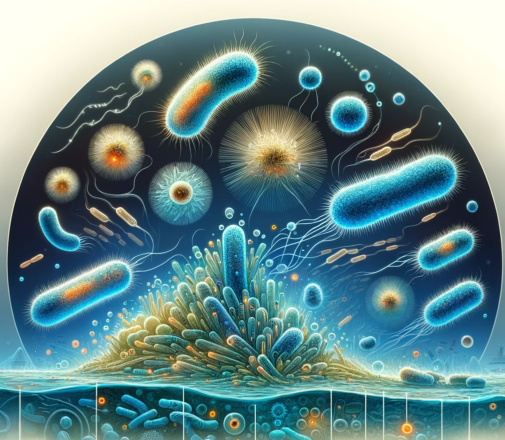
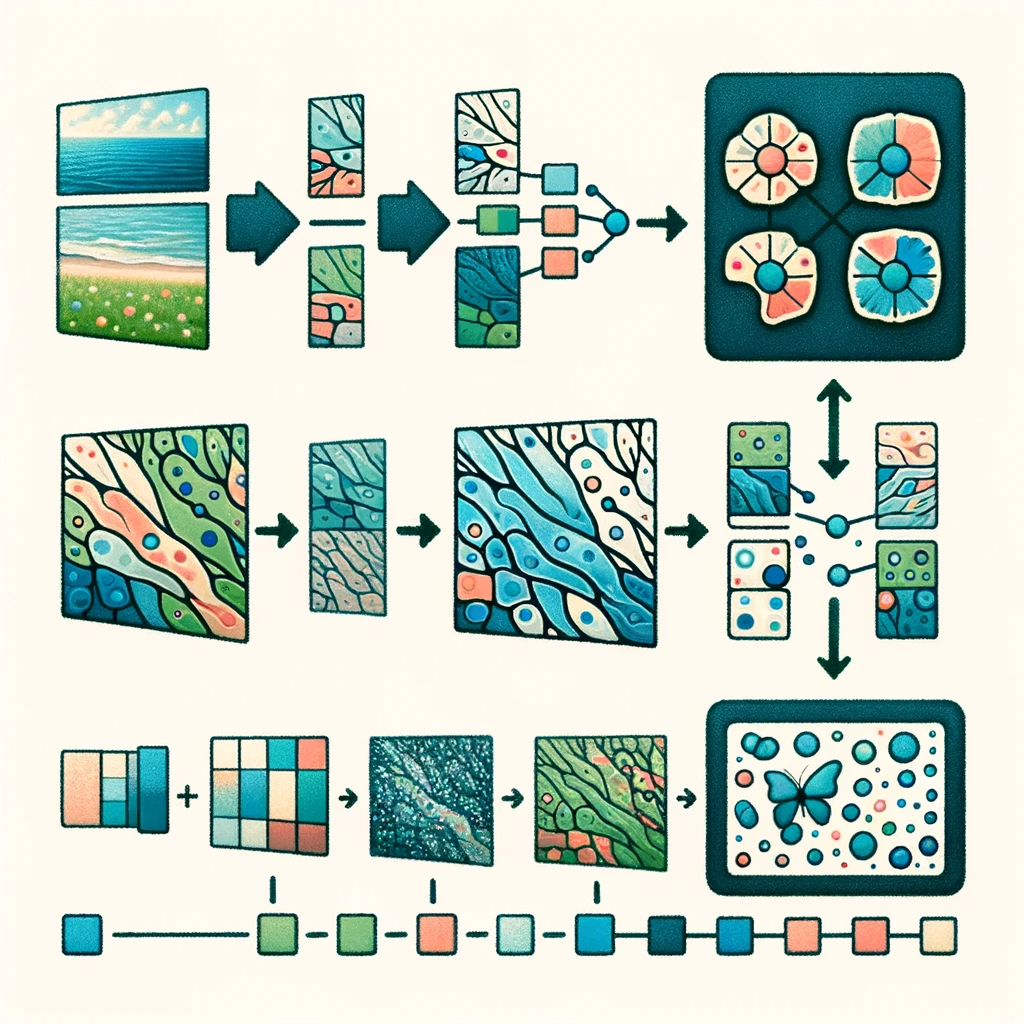
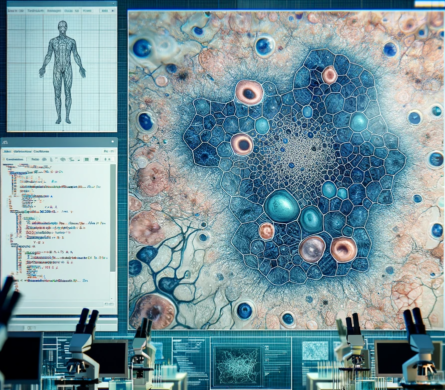

2018

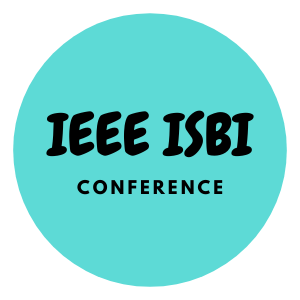
2017
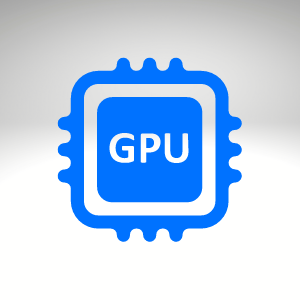
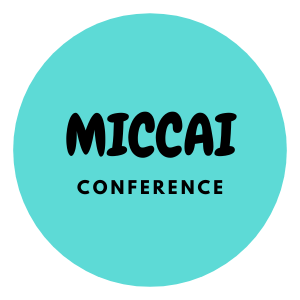
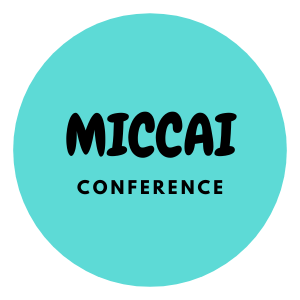
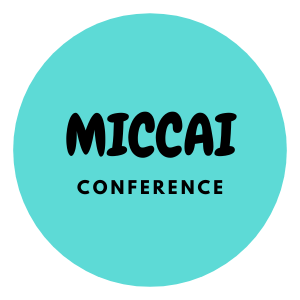
2016
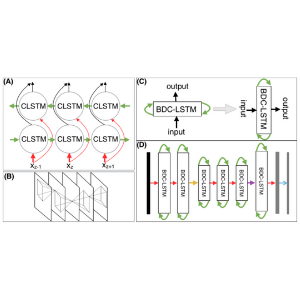
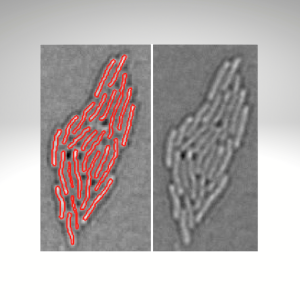
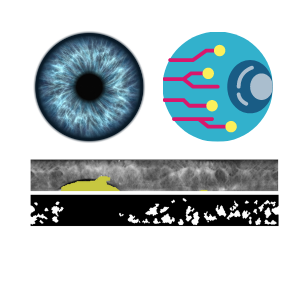
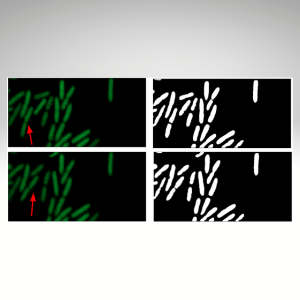
2015
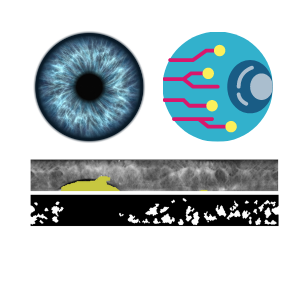
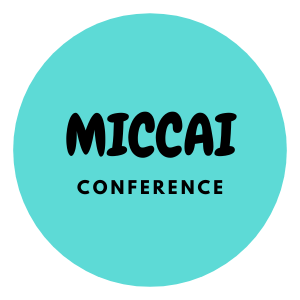
2014
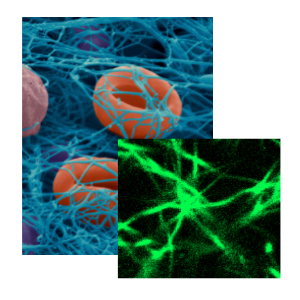
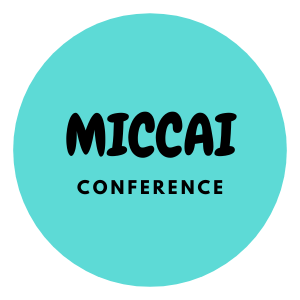